Focus Areas
We are creating new breakthroughs, fueling opportunity through exploration, and shaping history in real time.
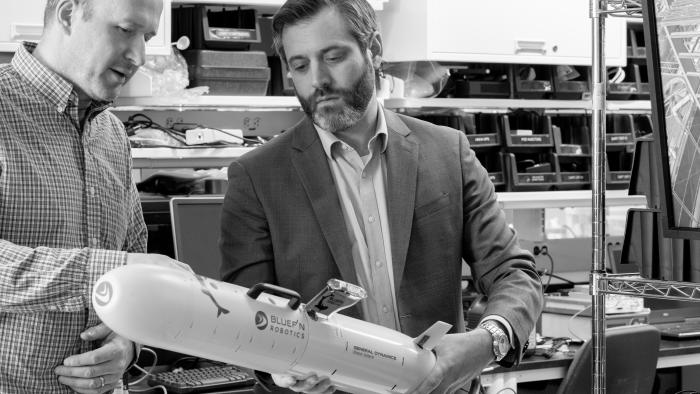
IMPACT REPORT
MITRE CONNECTS
MITRE accelerates progress when we connect the strengths of government, academia, and industry.
News & Insights
Acting as a bridge and convener to government, industry, and academia, MITRE delivers public interest impact to enhance the safety, stability, and well-being of our nation and the world. Follow our latest developments, stories, and technical resources.
Our Impact
Through objective insights, a unique vantage point, and technical know-how, we never stop discovering new ways to achieve results in the public interest.