Applying AI and machine learning in the aviation safety domain can lead to better decisions and continuous performance improvement.
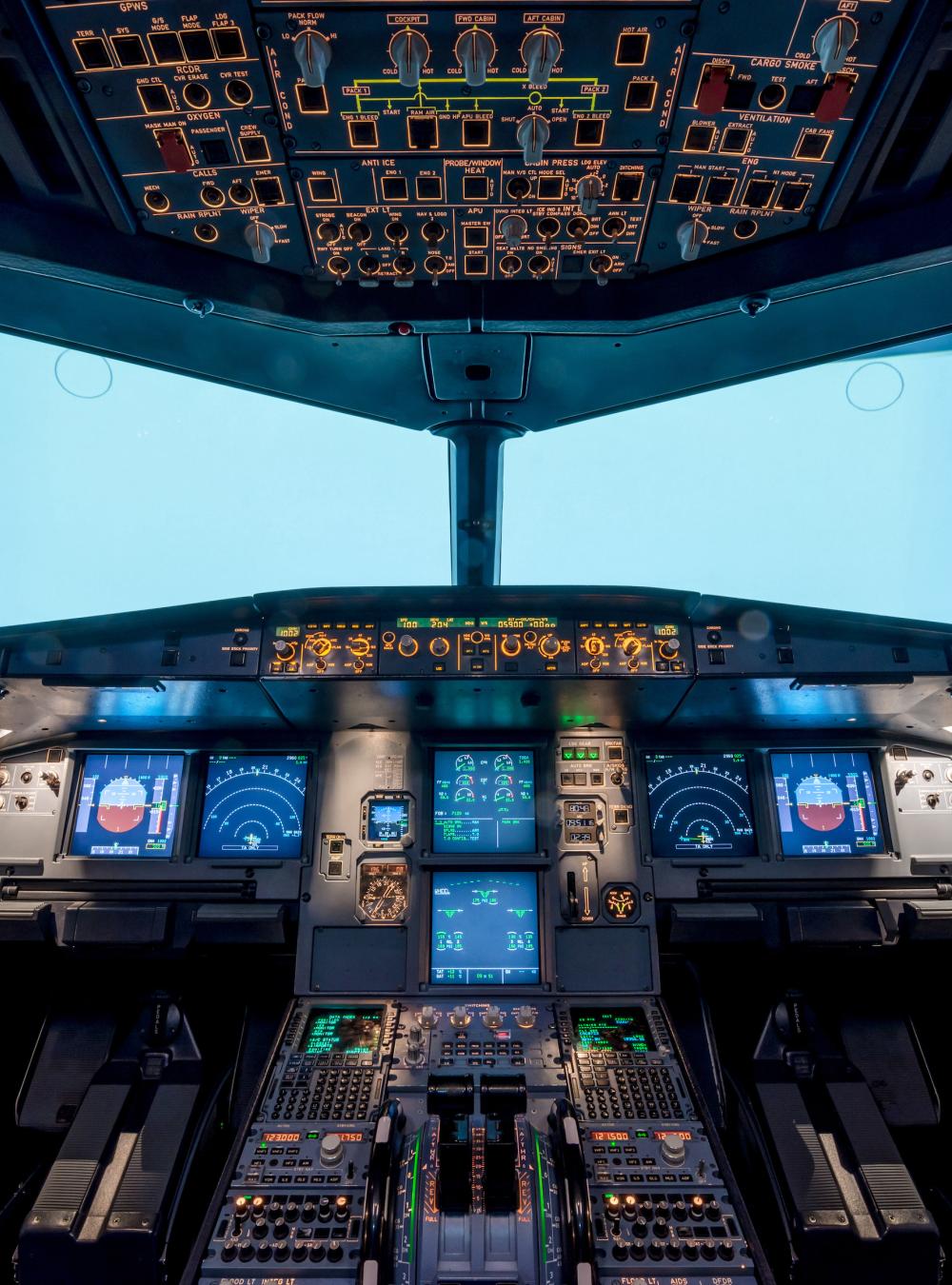
Using AI for Risk Discovery and Resolution in Aviation
What if the aviation industry could identify and address safety risks before they occur?
During the last 20 years, information sharing and collaboration between pilots, controllers, manufacturers, and maintenance personnel has led to major improvements in aviation safety. As the quantity of shared data has increased, artificial intelligence (AI) concepts and machine learning techniques needed to be introduced into complex, data-driven models to predict actions, recognize patterns, and uncover hidden insights.
MITRE’s application of AI helps our analysts recognize patterns in operational data, enhance analytics from multiple viewpoints, and connect data sets that provide insights at an extraordinary scale.
We're developing machine learning frameworks to explore risk identification and future methods to assist in identifying potential new or emerging safety issues, then evaluating and validating these frameworks. The efforts cut across surface, terminal, and en route operations to make aviation safer.
Data in a Haystack
"We de-identify, protect, and archive vast amounts of aviation safety data in a variety of types for each flight and from safety reports,” says Dr. Neeraj Koul, chief engineer of MITRE's Aviation Operations, Procedures and Safety Division.
"Due to the volume of disparate data, the challenge lies in evaluating and detecting unknown vulnerabilities and anomalies, as compared to known safety metrics."
Dr. Elizabeth Hohman, a chief data scientist in our Data and Human-Centered Solutions Technical Center adds, "Our engineers apply AI to automatically classify safety reports into known safety categories, identify reports similar to an exemplar report that contains an event of interest, or use numerical data to classify known undesired aircraft states."
One area where AI provides operational impact is its application to pilot safety reports.
"The manual exploration of hundreds of thousands of reports just isn’t feasible,” Hohman explains. “So, we apply a text classification technique to learn pilot safety report word and phrase patterns.
"As an example, it's very difficult to determine whether a flight operations quality assurance record has incorrect flap settings—since they can vary a great deal based on contextual factors such as weather and fuel burn—and human factors such as pilot preference and schedule.
"The text classification model identifies a much larger number of reports where pilots have manually recorded taking off with incorrect flap settings.”
Monitoring Air-Safety Trends Through Proactive Identification
Analyzing and understanding human factors (fatigue, workload, distraction) is a critical piece in improving aviation systems and safety standards.
And to break through the volume of safety reports, we implemented a semi-supervised text modeling method.
"This approach allows the analyst to use keywords to define topics of interest up front and influence the convergence of the model toward a result that reflects them," says Nick Kim, a senior data scientist.
"This added control provides a significant advantage over classical topic modeling approaches that define all topics.”
Text classification techniques are applied to monitor trends of previously known safety and human factors concepts. As safety analysts know what they're looking for, they can use machine learning to help sift through large amounts of text reports and find insights that will help them understand the factors that contribute to safety issues.
But what if we don't know what we’re searching for? As MITRE receives tens of thousands of new safety reports each month, using AI in text reports allows greater monitoring of new reported safety-related issues.
We use past reports as a baseline to identify new or surprising words or phrases. Comparing terminology and safety concepts from old reports with new allows proactive identification of potential safety hazards.
Deep Learning for Real-Time Results
What if something as humanly basic and innate as speech could be analyzed and used to improve aircraft safety?
MITRE has been developing automatic speech recognition techniques to convert Air Traffic Control (ATC) speech patterns into text, which can then be processed for post-operations analysis, real-time operations safety monitoring, and air traffic control training.
Aviation terminology is difficult for commercial-off-the-shelf products to translate accurately.
"Over the last several years, we've explored different AI techniques for extracting information from ATC voice communications," explains Hunter Kopald, principal systems engineer in our Aviation Concept of Operations and Research Division.
"As our dataset has grown, we’ve used more sophisticated techniques, like deep learning. We've trained our model on hundreds of thousands of real-time controller-pilot voice communications. The combination of data and deep learning has enabled a dramatic reduction in speech processing errors.”
Kopald and his team have also shown that these deep learning models can be used in near-real time. For example, current surface safety systems rely only on surveillance information to predict and alert for aircraft conflicts and collisions, which can lead to late or nuisance alerts.
Voice analysis of pilot/controller voice communication would provide information that, when combined with existing surface surveillance systems, could enable operators to detect potentially dangerous surface operations in real time—in some cases, much sooner than current systems can with surveillance data alone.
Pioneering Together for the Future of Aviation Safety
We still have work to do to connect the possibilities.
Can AI concepts that improve surface efficiency pioneer new advances in how we apply aviation safety data analytics?
Could ground-based speech recognition lead to alerting en route pilots of separation loss?
And, looking even further into the future, can AI be used to enable success as more new entrants enter the National Airspace System and improved surface-to-space architectures take shape?
MITRE is pioneering together with the FAA, industry partners, and academia to find the answers.
—by Matt Warnock
Note: This article originally appeared in a slightly different form in Managing the Skies Magazine, a publication of the FAA Managers Association.