MITRE’s data-driven responses to an NTIA inquiry requesting input into AI accountability policy development.
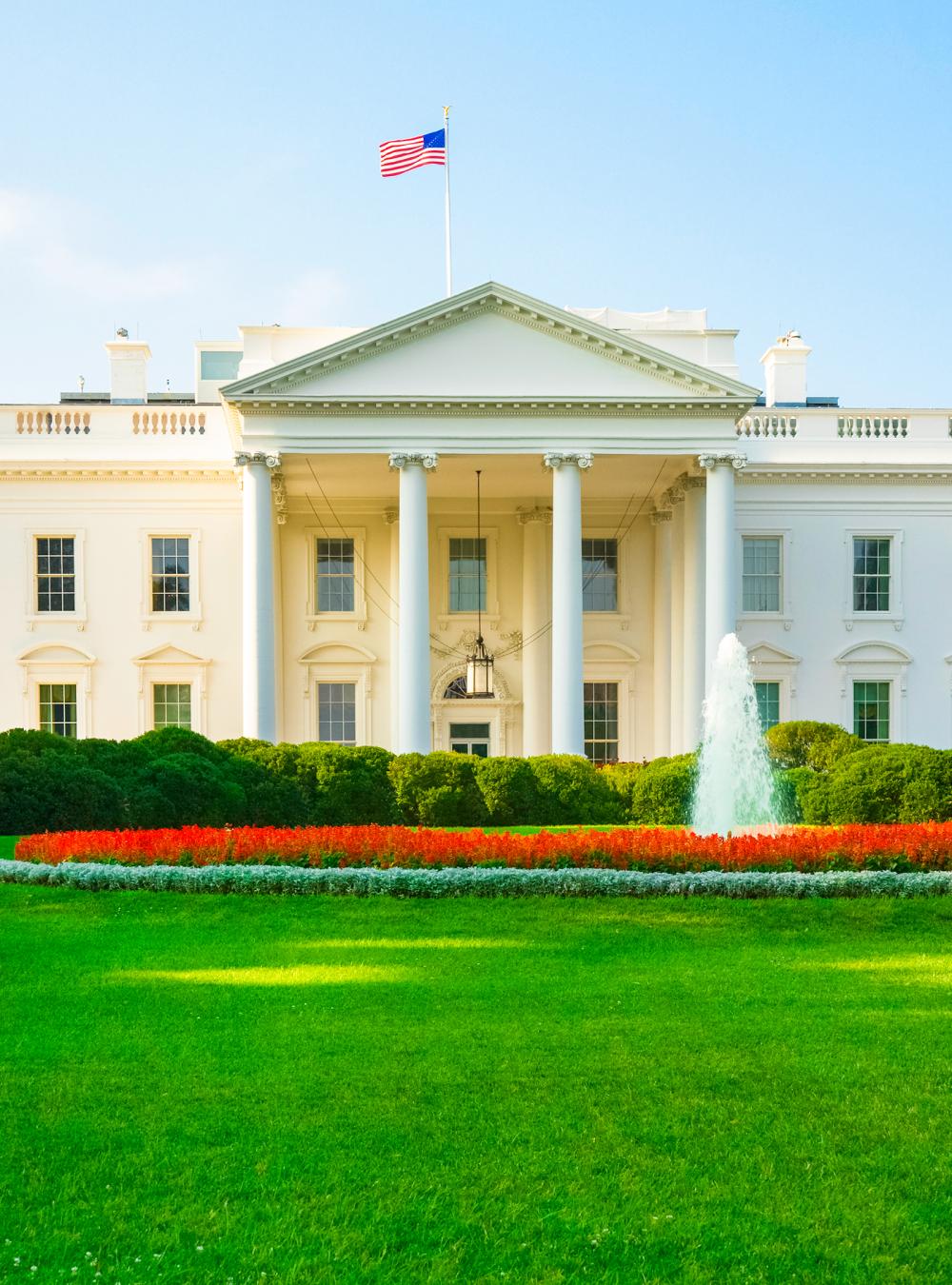
MITRE's Response to the NTIA RFI on Artificial Intelligence Accountability
Download RFI Response
What’s the issue? Advancing trustworthy artificial intelligence (AI) is an important federal objective with multiple supporting pieces of legislation and federal policy, including the National AI Initiative Act of 2020, the CHIPS and Science Act of 2022, a Blueprint for an AI Bill of Rights, and an AI Risk Management Framework. As the President’s principal advisor on telecommunications and information policy, NTIA issued a request for information to help ensure AI Assurance—that AI systems are legal, effective, ethical, safe, and otherwise trustworthy.
What did we do? The Center for Data-Driven Policy led a cross-MITRE analysis of NTIA’s posed questions to uncover data and evidence (from our work in the public interest) that would help them understand opportunities and develop plans that are evidence-based, actionable, and effective.
What did we find? AI growth has gone through tremendous technological advancement over the past six months, spawning a variety of recent policy proposals by various stakeholders on how to regulate AI. Some have been abstract and others targeted at specific considerations. Unfortunately, there isn’t an overarching framework on which to organize all these activities in a holistic, systematic manner. NTIA will thus need to conceptually map how its activities rely on and/or support external AI regulatory endeavors to achieve their desired impacts.
Within the AI security subdomain, any attempt to secure or regulate a new technology should be informed by vulnerabilities, threats that exploit those vulnerabilities (either intentionally or unintentionally), and the ultimate risk of damage, harm, or loss. This allows us to effectively model the threats and manage the risks. A complication for performing vulnerability, threat, and risk analysis for AI is that there is actually a wide range of algorithmic technologies within the AI umbrella, as well as a wide variety of dissimilar use cases.
AI assurance activities must also recognize the human component within the system. Human interplay within an AI lifecycle involves and impacts a broad range of stakeholders from end-users, developers, integrators, purchasers, deployers, and regulators to organizations and society. Therefore, AI assurance needs to be conceptualized and implemented in a sociotechnical context throughout system development, verification, deployment, and governance—all of which will vary by use case. Individuals leveraging AI must also be accountable for proper usage of its output and should face consequences if they’re careless.