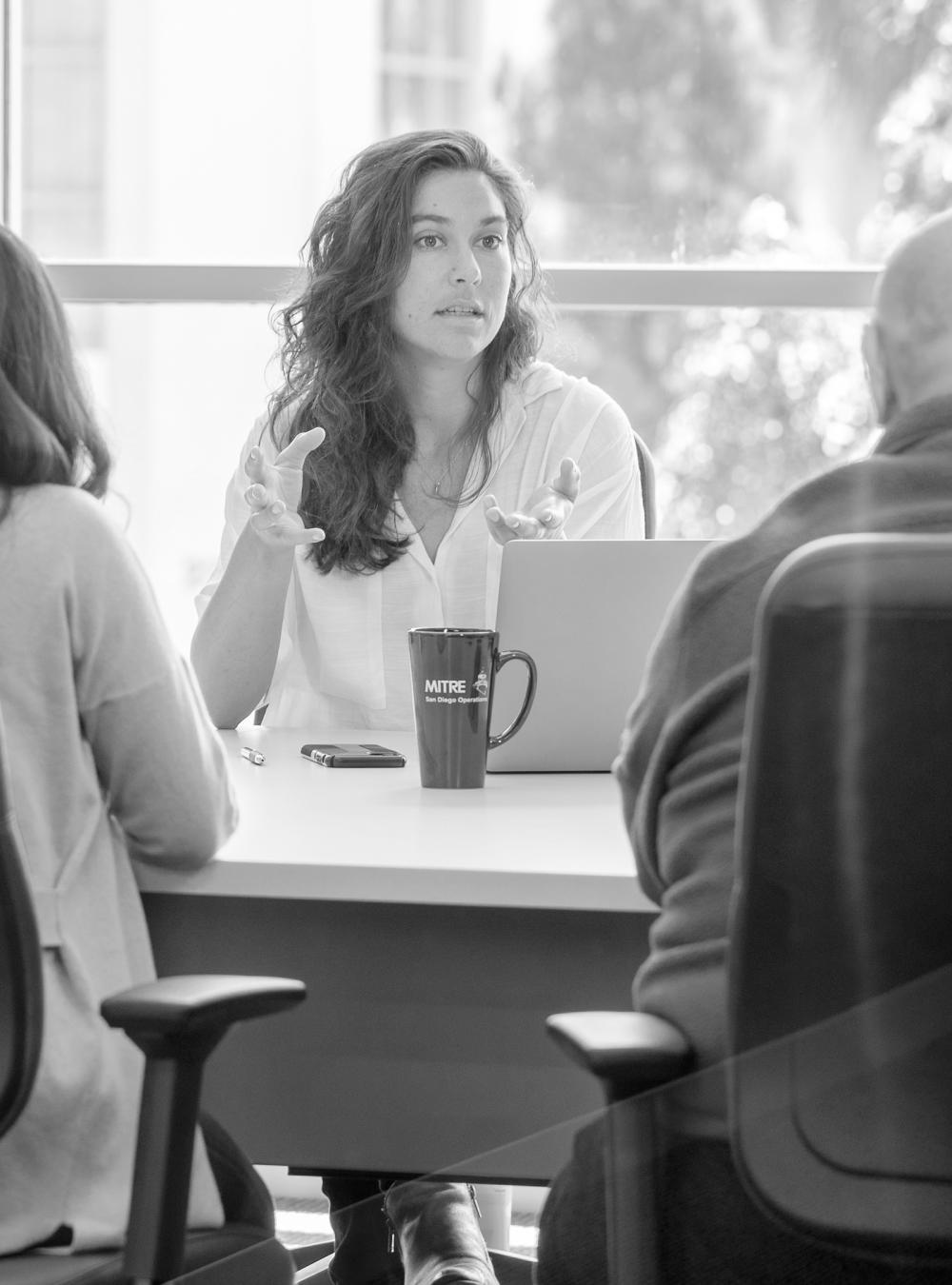
News & Insights
Follow our latest developments, stories, and technical resources.
Media Inquiry
Reach out to us for media requests and public inquiries regarding MITRE—our work, our executive team, and our technical experts.
Events
View our noteworthy events either hosted by MITRE or where company representatives are active participants.