This paper provides a systems approach to addressing attacks, consequences, and mitigations for Machine Learning explained over its lifecycle.
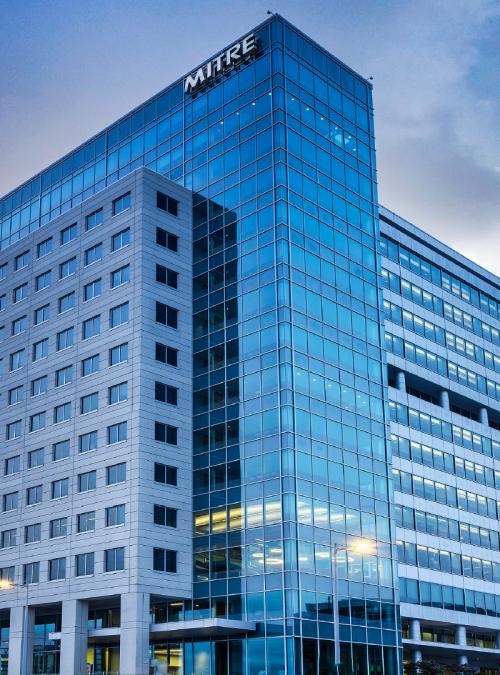
Odyssey: A Systems Approach to Machine Learning Security
Download Resources
Using software exposes vulnerabilities that are susceptible to attacks with serious consequences. While there are many different vulnerabilities, the consequences fall into a small number of categories. This paper explains how consequences of attacks on Machine Learning (ML) vulnerabilities fall into these same categories. These consequences are then aligned to ML-specific attacks, the contexts in which they occur, and the established methods for mitigating them. These defensive countermeasures can support the security of the ML elements of a system and lead to greater assurance that systems using ML will operate as planned.
This paper provides a systems approach to addressing attacks, consequences, and mitigations for systems using ML. It explains each of these over the lifecycle of an ML technology, providing clear explanations of what to worry about, when to worry about it, and how to mitigate it while presuming little incoming knowledge of ML specifics.