MITRE and the Hospital for Special Surgery collaborated to create an artificial intelligence-driven toolkit to facilitate faster, more accurate diagnoses of arthritis. The technology can help improve treatment and opens doors for advances in rheumatology research—and potentially for other diseases.
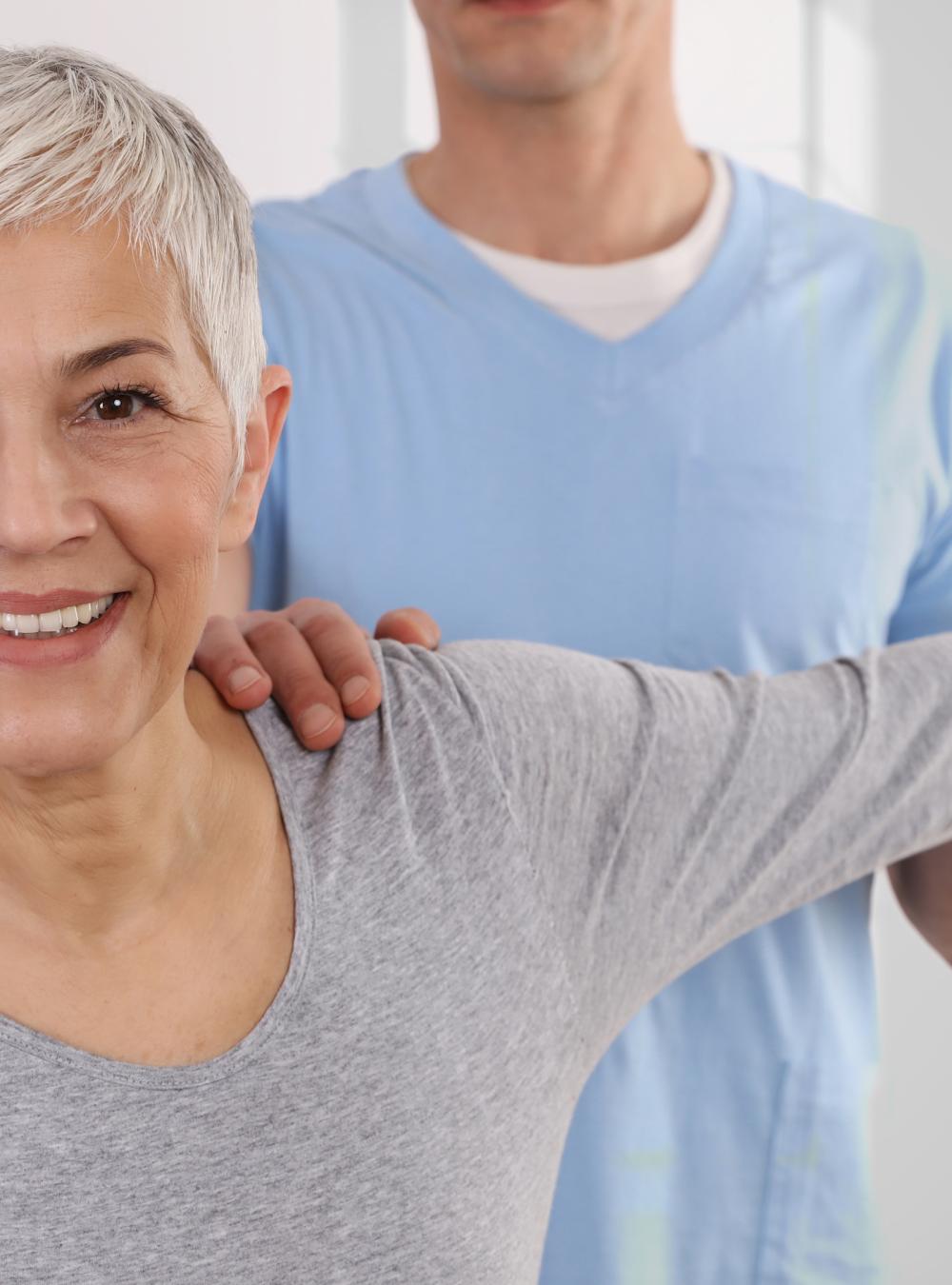
Reducing the Ambiguity Around Arthritis: AI-Driven Diagnostics to Improve Patient Outcomes
Rheumatoid arthritis and osteoarthritis affect more than 4 million Americans annually. Inflammation in the joint can cause pain, discomfort, and sometimes debilitating symptoms.
Both conditions impact patients, and respond to treatments, very differently. Successful care depends on distinguishing not only between the diseases, but within them.
Rheumatology arthritis research in particular strives to tailor treatments to patients by determining the type of inflammation in their joints, a.k.a. the disease subtype. These discrete subtypes respond differently to various treatments. And currently, identifying subtypes requires expensive testing that doesn’t always deliver accurate results. The ambiguity carries big implications for arthritis patients: A mere 40% improve by just 50% with any given treatment.
To decrease uncertainty and improve outcomes, MITRE collaborated with New York’s Hospital for Special Surgery (HSS) and other partners to apply computer vision and AI tools to aid in disease diagnosis and subtype classification.
This kind of collaborative research exemplifies how merging ideas and expertise from traditionally disparate fields can improve the health and wellness of millions of people.
Under our independent R&D program, a collective research team created a novel approach that improves on existing open-source algorithms used in histopathology, the study of patient joint tissue samples. MITRE’s histological AI toolkit can assist and augment expert analysis by automating labor-prohibitive tasks—like counting 100,000+ nuclei in a digital image of a tissue sample.
For people suffering from rheumatoid arthritis, the innovation can deliver quicker, less expensive, more reliable diagnoses. That opens the door to better treatment options, relief from chronic pain, and improved patient care and wellbeing.
“We’re excited to see how this initiative unfolds,” says Dr. Dana Orange, HSS assistant attending rheumatologist, who facilitated coordination between clinical experts and MITRE engineers. “We believe it can enable computer-automated histopathology to provide a level of precision, reproducibility, and scale that are just not possible for even the best human pathologists to achieve on their own.”
Sights on the Stars to Improve Patient Care
Since the days of looking at slides on a microscope, the pathology field has evolved considerably. Today’s digital imaging points cameras at the tissue sample and converts it to a whole-slide image. Computer vision helps identify objects in that image, as well as understand what the image depicts.
But these images are massive—upwards of a billion pixels—like looking at a billion stars. As a result, current software allows analysis of a whole-slide image, but in an extremely cumbersome way.
“Pathologists have to point and click and drag things around on this huge image to try to find what they’re looking for,” says MITRE’s James Thompson, the project’s co-principal investigator. “It’s better than looking at a slide on a microscope, but the field hasn’t really modernized how the data gets analyzed.”
The highly manual process introduces a certain degree of subjectivity, which can impact treatment decisions. For example, one pathologist may analyze a tissue sample and determine it “looks angry,” meaning the arthritis is worsening. But another pathologist could examine the same sample and come to a different conclusion.
To tackle the challenges of scale and subjectivity, our researchers explored the parallel between galactic images and images of tissue samples.
“Many satellite images are on the order of a billion pixels,” Thompson explains. “So there’s a direct translation between looking at the microscale of cell nuclei inside human tissue to looking at the macroscale of points on the surface of the Earth.”
The correlation prompted an interesting question: How could we apply MITRE’s aerospace expertise to improve arthritis diagnosis?
Our AI experts worked with our aerospace engineers to apply novel astronomy-based approaches to the problem. The research team developed an objective, standardized approach based on quantifiable metrics. The algorithms leverage machine learning to enable pathologists to see at low and high resolution—and take in the global picture.
In the short term, patients will more likely get the same results from different doctors. For the long run, the approach supports the ongoing study of distinct disease characteristics, as well as causes and correlations.
“That should lead to better research and push advances in the field,” says MITRE’s David Slater, co-principal investigator. “Now we can compare apples to apples across separate experiments. A repeatable process means anyone examining any image can come to the same numerical values and outcomes every time.”
The process also required a well-designed data system that can facilitate users on multiple scales. MITRE developed an open-source pipeline that allows each whole-slide image to be associated with relevant patient information, clinical observations, and other data to aid in analysis. Additionally, it enables storing those images, on Amazon Web Services or another publicly available cloud system, so users can easily access them.
Empowering—Not Supplanting—Human Experts
Despite AI’s powerful potential, pathologists and rheumatologists needn’t worry about job security. Rather than replace these specialists, the toolkit quickly and accurately tells them where to look, ensures attention to all those locations, and quantifies features once only qualitatively assessed.
For pathologists, this can dramatically change their workflow and provide critical additional diagnostic information.
“The more quantification and standardized measurement we can put to something, the more certain we can be of diagnosis and understand what causes the disease,” Thompson says. “That can open the aperture to future research as to how to treat the disease before it manifests symptoms and how to detect it earlier.”
In certain cases, the technology could also assist non-expert analysis and diagnosis. For example, the algorithms could be deployed to remote clinical locations (e.g., a military field hospital or rural site) staffed by general practitioners.
To provide insights for additional research in rheumatology, the research team shared their findings in the Journal of Arthritis Research and Therapy, ACR Open Rheumatology, and Arthritis and Rheumatology. We anticipate the innovation could expand to support other disease diagnoses, as well.
The initiative, which grew from connections through other MITRE collaborations, draws on our research on applying AI in clinical settings. These include efforts with Boston Children’s Hospital to reduce risk in pediatric catheterization, with the Ottawa Heart Institute for cardiac procedures, and with Rockefeller University for RNA studies.
Moving forward, we’re tapping into our role as operator of six federally funded R&D centers to explore other potential use cases for our government sponsors. We’re also developing a technology transition plan, as part of our commitment to share our research and the resulting capabilities with industry for the public good.
“This kind of collaborative research exemplifies how merging ideas and expertise from traditionally disparate fields can generate better diagnoses—and improve the health and wellness of millions of people,” Thompson says.
Join our community of innovators, learners, knowledge-sharers, and risk takers. View our Job Openings.