In this Q&A, two MITRE researchers discuss how innovations in technology can support the physical and mental health of our nation's veterans.
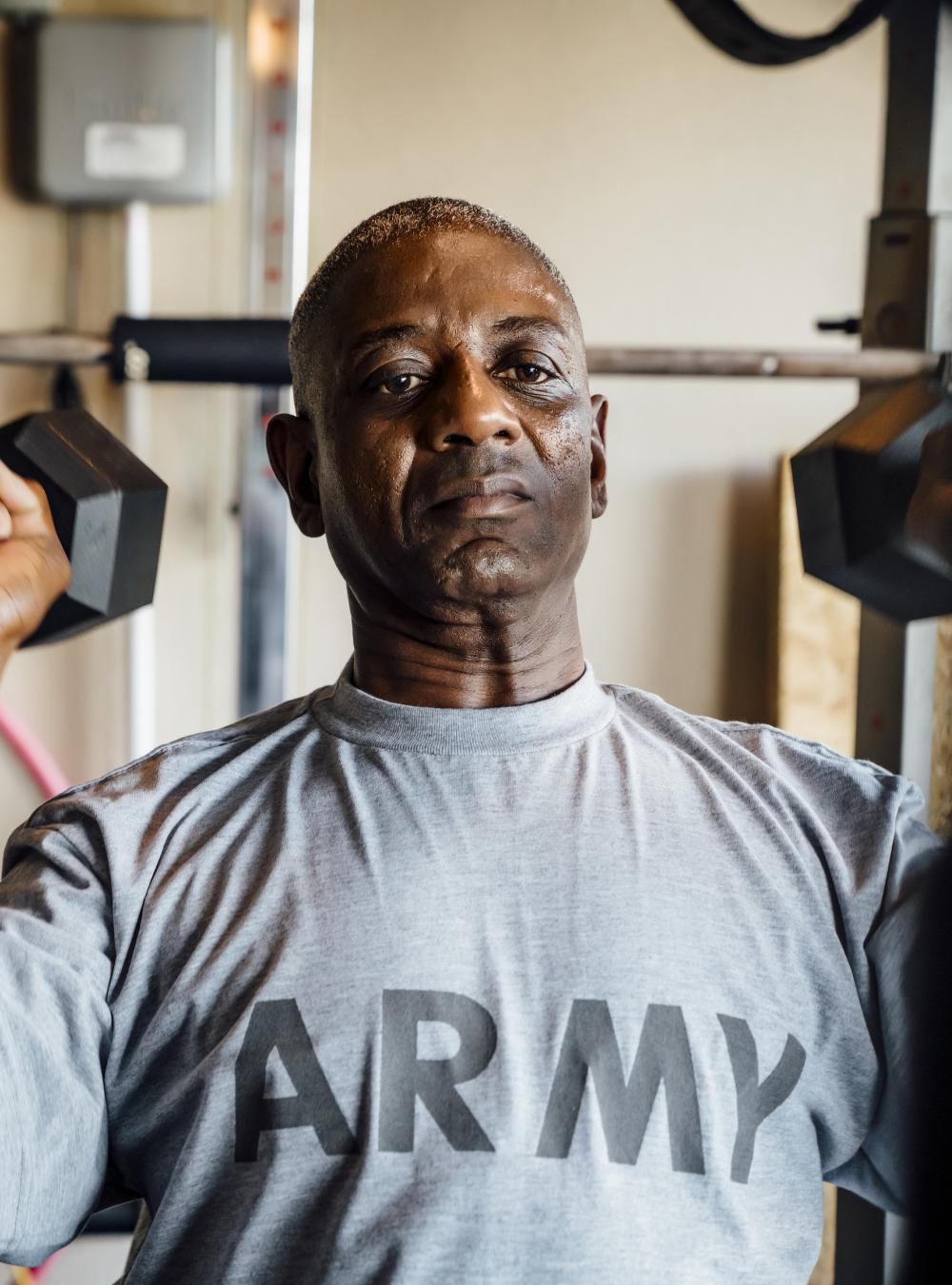
Using Artificial Intelligence to Improve Health Outcomes for Veterans
Sanith Wijesinghe is the innovation area lead for modeling, simulation, experimentation, and analytics. His work has explored ways of increasing compliance and reducing fraud in tax and benefits delivery, among other topics. In March, we spoke with Wijesinghe about his work using data to improve health and benefits delivery for veterans. We recently talked with Wijesinghe again, along with Dr. Sybil Klaus, who leads MITRE's health innovation research, to understand more about how MITRE is using artificial intelligence (AI) to address a range of tough challenges in the veteran community.
Can you give us a sense of how MITRE looks at AI as a way to solve problems in healthcare and service delivery for veteran populations?
Sanith Wijesinghe: We really begin with a systems approach. Patients and hospitals are components of complex systems where outcomes are driven by the interaction of many other interwoven systems. As such, classical statistical analysis can be misleading or inconclusive because the underlying assumptions of these methods may not be true.
Right now, we’re partnering with several hospitals and universities to research and develop AI solutions to clinical problems.
Sybil Klaus: One example is a study with Boston Children’s Hospital. MITRE has been exploring the viability of an alternative statistical approach called multivariate-multifractal analysis to detect adverse patient events including central line-associated bloodstream infections in patients.
These can occur when a patient has a catheter in a large vein to draw blood or give fluids. Since central lines often stay in place for a long time, bacteria sometime enter the bloodstream and cause an infection.
SW: Our study fuses multiple physiological data streams together to measure the global state of a patient and find signatures of adverse health events before they occur. We also fuse administrative and clinical data to measure the global state of the system—in this case, a hospital—to better understand how the system can mitigate and respond to potential adverse events. That would be difficult to do with the standard approach.
Where are some areas within the arena of veterans’ health where AI can help bring about better outcomes?
SK: We're interested in mental health as a broad area for improving patient outcomes. Mental disorders—including serious mental illness, such as schizophrenia or bipolar disorder, depression, anxiety disorders, and substance use disorders—are particularly acute within the veteran community where elevated rates of post-traumatic stress disorder and traumatic brain injury have led to an epidemic of mental health issues.
SW: We know that early intervention is essential to saving lives. That’s why we're exploring whether AI techniques, fine-tuned to detecting anomalies in physiologic variables from wearable sensors, could indicate disease onset.
Given the COVID-19 crisis, are there opportunities to use AI to support veterans in getting care?
SK: The CARES Act was signed in late March, and one of its features is support for veterans. The CARES Act specifically calls upon the VA to help expand “telemental health services for isolated veterans.” In our view, this creates a real need for demand forecasting for mental health services. That way, you can align staff resources efficiently.
SW: We approach it with a combination of data analytics, modeling, and simulation tools to allow decision makers to explore “what-if?” scenarios. It’s important because when you're developing a nationwide response—across many different urban, suburban, and rural communities—the solutions we offer should take into account dependencies at scale.
SK: With that kind of problem, AI techniques help us explore alternatives to help match supply and demand that may not have been considered otherwise.
How does MITRE approach issues of privacy and fairness in the design and use of AI in the health arena?
SW: AI techniques typically require significant amounts of “ground truth” data to converge on accurate predictions, which can be a challenge in the health arena. Now, however, there are classes of “generative” methods gaining traction that don't have this pre-requisite.
Generative methods create realistic data that modelers can work with. A prime example of this is our Synthea data generation and disease modeling tool, which has been widely accepted in the health IT community.
SK: With Synthea, researchers get the vast amount of data they need to conduct health IT development and experimentation.
That allows them to put the power of AI technology to use for without compromising patient privacy.
—Interview conducted by Greg Michaelidis