AI is great at finding more of what you know–but not at finding things you never thought to look for. Let’s change that.
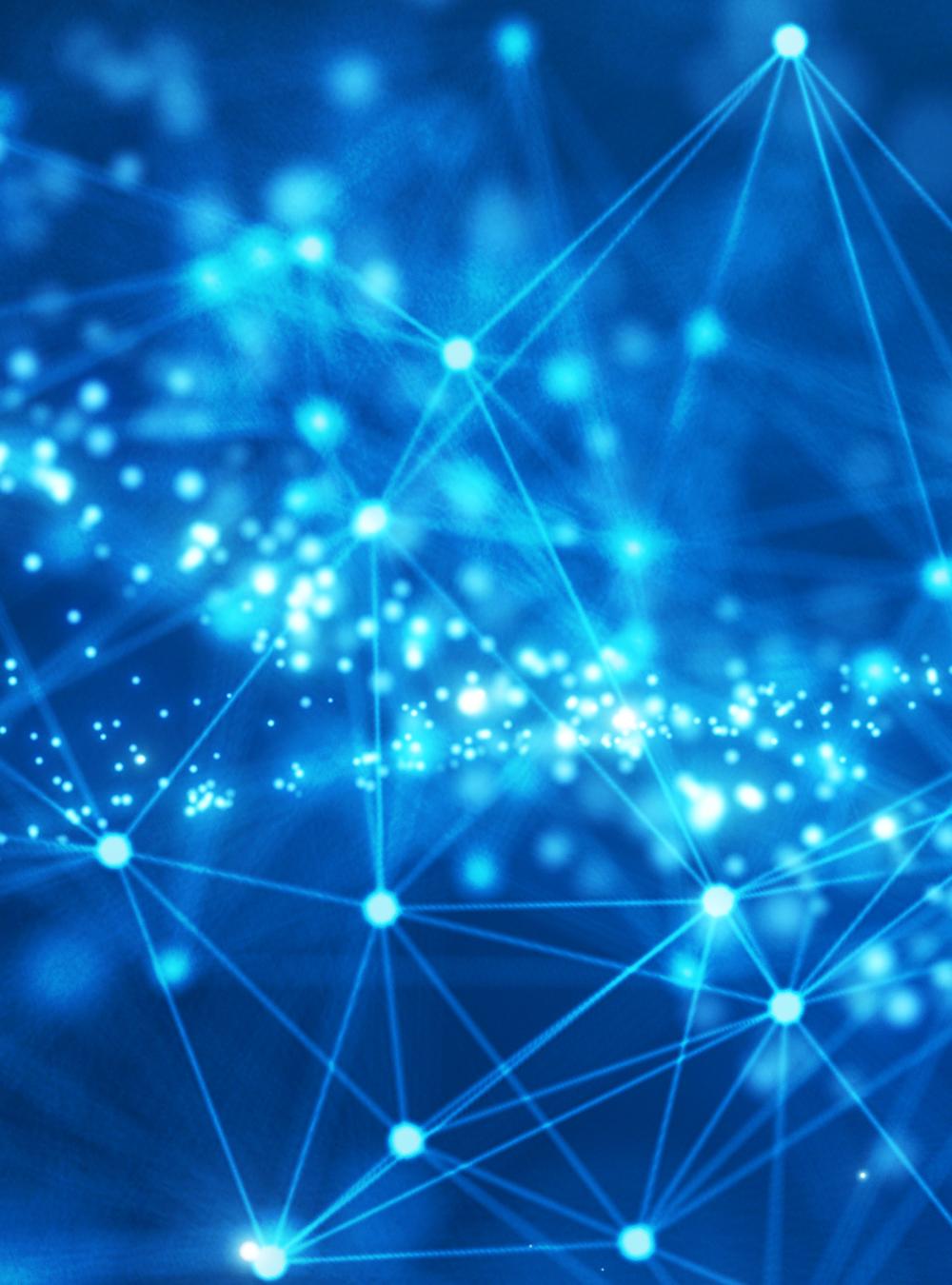
Intelligence After Next: Breaking Past AI’s Confirmation Bias
Download Resources
Investigating alternate hypotheses is an important part of both science and intelligence analysis. Confirmation bias pushes us to overweight evidence matching our initial hypothesis and discounting evidence to the contrary. Intelligence analysts know not to cling too tightly to a hypothesis until they acquire enough evidence to support it and find no or little evidence that contradicts it. Current artificial intelligence (AI) systems help us with finding supporting evidence but does little to help us find contradictory evidence.
AI excels at helping analysts automate the task of finding results in a vast sea of data based on previously known examples. It can locate heavy equipment in overhead imagery, identify documents of potential interest out of millions of others, and sort photos into like bins. It is more difficult for AI to help us find things we do not know to look for as AI applications often have the same built-in confirmation bias we try to avoid. We need systems that not only help us find supporting evidence but also potential evidence for one or more alternate hypotheses.
Two potential paths using AI applications can help analysts investigate alternate hypotheses more thoroughly. These include:
- “Widening the net” by using an ensembled approach of machine learning algorithms for clustering, classification, and word embedding to identify topics outside an initial investigation.
- Augmenting analytical structured argumentation with information retrieval systems designed to help analysts locate information supporting or contradicting their initial hypotheses.
In addition, future investments are necessary to make the process of identifying alternate hypotheses more valuable to analysts. These include providing better tools to help analysts organize their evidence and, at the same time, identify potential gaps in their arguments; building in features to highlight the analysis of competing hypotheses into search engines; and identifying ways in which AI can assist analysts in filling out a structured argument pathway or searching for evidence to support each node in their argument.
AI can play an important role in strengthening the use and effectiveness of alternate hypotheses in the analytic process, leading to stronger and more accurate assessments for the IC’s current and future customers.